Data Science is the art of turning data into actions. This is accomplished through the creation of data products, which provide actionable information without exposing decision makers to the underlying data or analytics (e.g., buy/sell strategies for financial instruments, a set of actions to improve product yield, or steps to improve product marketing).
Performing Data Science requires the extraction of timely, actionable information from diverse data sources to drive data products. Examples of data products include answers to questions such as: “Which of my products should I advertise more heavily to increase profit? How can I improve my compliance program, while reducing costs? What manufacturing process change will allow me to build a better product?” The key to answering these questions is: understand the data you have and what the data inductively tells you.
Data Product
A data product provides actionable information without exposing decision makers to the underlying data or analytics. Examples include:
- Movie Recommendations
- Weather Forecasts
- Stock Market Predictions
- Production Process Improvements
- Health Diagnosis
- Flu Trend Predictions
Background:
The term Data Science appeared in the computer science literature throughout the 1960s-1980s.
It was not until the late 1990s however, that the field as we describe it here, began to emerge from the statistics and data mining communities. Data Science was first introduced as an independent discipline in 2001. Since that time, there have been countless articles advancing the discipline, culminating with Data Scientist being declared the sexiest job of the 21st century.
What makes Data Science different?
Data Science supports and encourages shifting between deductive (hypothesis-based) and inductive (pattern-based) reasoning. This is a fundamental change from traditional analytic approaches. Inductive reasoning and exploratory data analysis provide a means to form or refine hypotheses and discover new analytic paths. In fact, to do the discovery of significant insights that are the hallmark of Data Science, you must have the tradecraft and the interplay between inductive and deductive reasoning. By actively combining the ability to reason deductively and inductively, Data Science creates an environment where models of reality no longer need to be static and empirically based. Instead, they are constantly tested, updated and improved until better models are found. These concepts are summarized in the figure below:
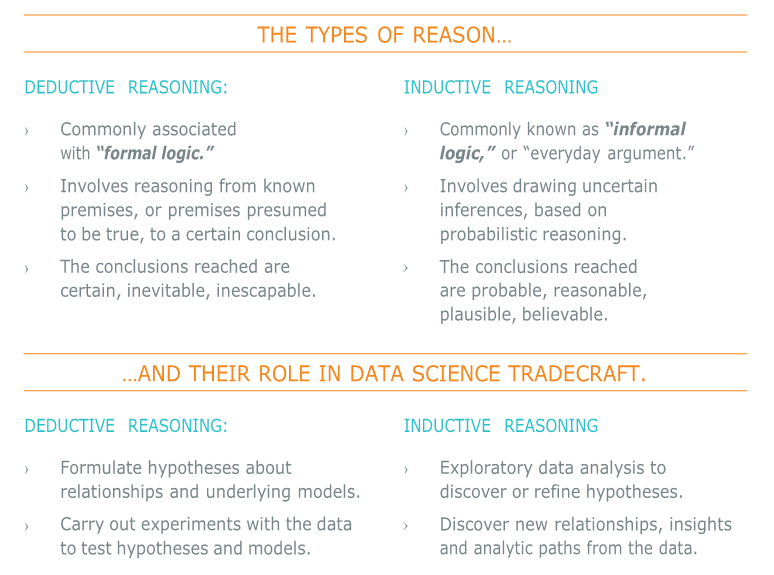
The differences between Data Science and traditional analytic approaches do not end at seamless shifting between deductive and inductive reasoning. Data Science offers a distinctly different perspective than capabilities such as Business Intelligence. Data Science should not replace Business Intelligence functions within an organization, however. The two capabilities are additive and complementary, each offering a necessary view of business operations and the operating environment.
The figure, Business Intelligence and Data Science – A Comparison, highlights the differences between the two capabilities. Key contrasts include:
Discovery vs. Pre-canned Questions: Data Science actually works on discovering the question to ask as opposed to just asking it.
Power of Many vs. Ability of One: An entire team provides a common forum for pulling together computer science, mathematics and domain expertise.
Prospective vs. Retrospective: Data Science is focused on obtaining actionable information from data as opposed to reporting historical facts.
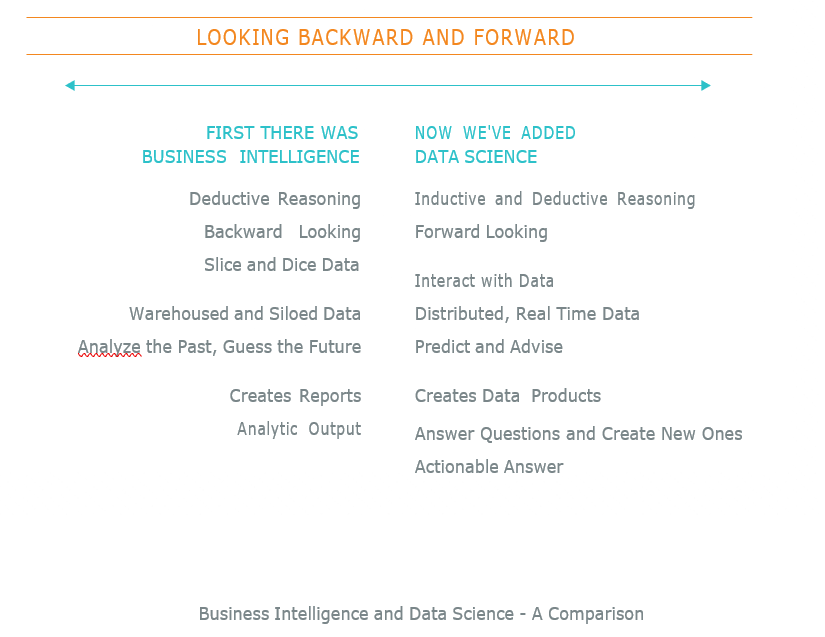
Source: Booz Allen Hamilton
Leave feedback about this